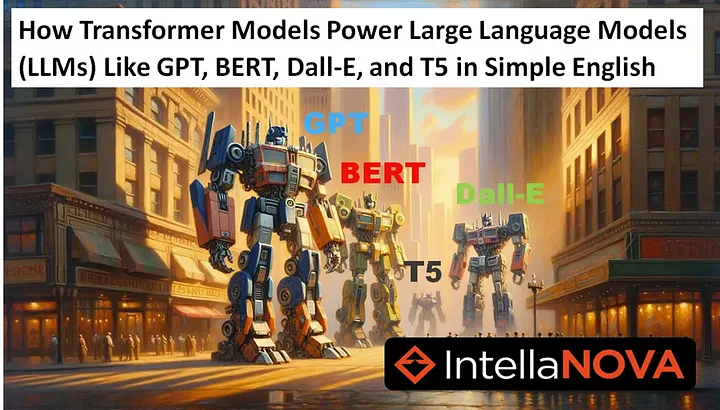
Transformers: The Past, Present, and Future of Artificial Intelligence
The field of artificial intelligence (AI) has seen profound transformations in recent years, and one of the most significant drivers of this change is the Transformer architecture. Originally introduced in the 2017 paper Attention Is All You Need by researchers at Google Brain, Transformers have revolutionized how AI models understand and process data. These models have empowered advancements across various domains, from conversational agents like GPT-3 to state-of-the-art image recognition systems and even autonomous vehicles. However, while Transformers have unlocked remarkable potential, they are far from perfect, and there are several key challenges that need to be addressed for them to realize their full potential.
In this article we will explore the evolution of Transformers, their current limitations, and the exciting innovations that may shape the next phase of AI.
Transforming the AI Landscape
PAST: Before the advent of Transformers, machine learning models for tasks like natural language processing (NLP) relied heavily on recurrent neural networks (RNNs) and long short-term memory networks (LSTMs).
CHALLENGE (RNN): These architectures struggled with understanding long-range dependencies in data due to their sequential processing nature.
PRESENT: The introduction of the Transformer, with its self-attention mechanism, changed everything.
DIFFERENTIATION (Transformer): By allowing models to consider all input tokens simultaneously, Transformers are capable of capturing complex, contextual relationships within the data without being hindered by sequential order.
USECASE: This breakthrough laid the foundation for models like:
- OpenAI’s GPT-3, a language model that can generate human-like text, DALL-E 2, which creates images from text prompts
- Vision Transformers (ViTs), which have redefined image classification tasks.
APPLICATION: These innovations have made Transformers a central force in AI, with applications across healthcare, finance, entertainment, and autonomous systems.
Despite their success, several critical limitations remain.
The Current Limits of Transformers
While Transformers have significantly advanced AI, there are several challenges that continue to constrain their widespread adoption:
CHALLENGE #1 — Compute-Heavy: One of the most obvious limitations of Transformers is their massive computational requirements. Training models like GPT-3 requires an astronomical 3,640 petaflop/s-days of computational power, a figure that has only grown with newer models like GPT-4. The computational intensity of training these models means that only a few well-funded organizations have the resources to build them, exacerbating concerns about accessibility.
CHALLENGE #2 — Data Dependency: Transformers are highly reliant on large datasets to perform well. However, obtaining vast amounts of high-quality data is not always possible, especially for specialized domains or underrepresented languages. This creates a significant barrier to entry for smaller companies or industries that require tailored AI solutions.
CHALLENGE #3 — Bias and Ethics: Transformers are trained on datasets that often reflect societal biases, and as a result, these models can inadvertently perpetuate harmful stereotypes or generate unreliable outputs. This is particularly concerning in sensitive applications like hiring, lending, or law enforcement, where biased predictions can have serious real-world consequences.
CHALLENGE #4 — Interpretability Issues: One of the most pressing concerns with Transformer models is their lack of interpretability. The “black-box” nature of these models makes it difficult to understand how they arrive at specific decisions. In industries like healthcare and finance, where model predictions can significantly impact people’s lives, the inability to explain these decisions is a major roadblock.
Opportunities for Innovation
FUTURE: Despite these challenges, the future of Transformer models looks promising. Several areas of innovation offer hope for overcoming these limitations and unlocking new possibilities:
- Architectural Adjustments: Techniques like sparse attention, which reduces the number of tokens the model needs to focus on, and memory-augmented networks, which can retain information over longer sequences. Both of these promises to make Transformers more efficient and scalable. These advancements could reduce computational costs and improve the model’s ability to process longer data sequences.
- Democratization of AI: To address the issue of accessibility, the AI community is exploring model distillation and federated learning. Distillation allows large models to be “compressed” into smaller. This allows transformers to be more efficient without losing significant performance. Federated learning enables organizations to train models collaboratively while keeping data decentralized, which could make powerful AI tools accessible to smaller enterprises and reduce concerns over data privacy.
- Sustainability Initiatives: As the environmental impact of AI models becomes more apparent, there is a growing push to reduce the carbon footprint of training and deploying large models. Optimizing model architectures to use less energy and employing renewable energy sources in data centers are two ways that the AI community can mitigate the environmental impact of Transformers.
- Bias Mitigation: One of the most critical areas for improvement is bias mitigation. Researchers are exploring ways to curate more ethical datasets and develop fairness-focused training algorithms. By addressing biases at the data and algorithmic levels, we can ensure that AI models are more equitable and inclusive.
Conclusion: A Collaborative Effort for a Transformative Future
As we look toward the future of Transformer models, it’s clear that there is tremendous potential for further breakthroughs. However, to realize this potential fully, the AI community must work collaboratively across academia, industry, and government to address the ethical, computational, and interpretability challenges that remain. Innovations in model architecture, accessibility, sustainability, and bias mitigation are all promising avenues that will help shape the next phase of AI development.
By working together to overcome these hurdles, we can ensure that Transformer models continue to advance AI in a responsible and inclusive way, unlocking new opportunities across industries and ultimately improving the lives of people around the world. The road ahead is exciting, but it will require collective action and a commitment to solving the challenges that stand in the way of a more accessible, ethical, and sustainable AI future.