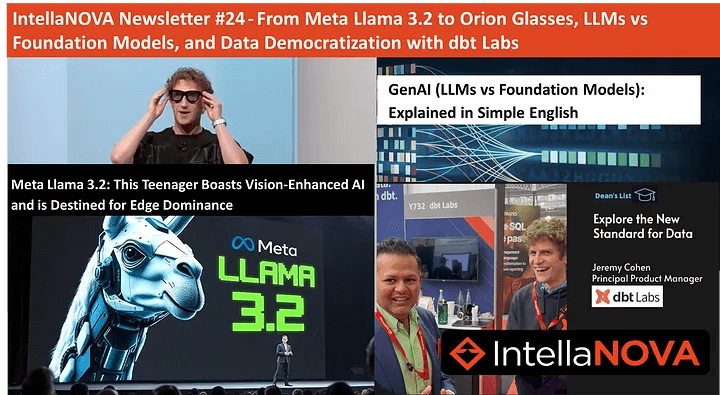
IntellaNOVA Newsletter #24 — From Meta Llama 3.2 to Orion Glasses, LLMs vs Foundation Models, and Data Democratization with dbt Labs
Meta Llama 3.2: This Teenager Boasts Vision-Enhanced AI and is Destined for Edge Device Dominance!
At Meta Connect, Meta unveiled Llama 3.2, a major advancement in AI that introduces vision capabilities alongside text-based intelligence, making it a significant upgrade from its predecessor.
Llama 3.2 offers new model sizes, including 11 billion and 90 billion parameter versions that are drop-in replacements for Llama 3.1. Llama 3.2 enables users to access both text and vision-based intelligence without overhauling existing code. Additionally, smaller models optimized for edge devices, like smartphones and IoT, are also available. These smaller models feature 128k context windows ideal for summarization and text generation.
Llama 3.2’s vision models excel in image reasoning tasks such as graph comprehension and image captioning, while the open-source Llama stack toolkit supports flexible deployments across cloud and edge environments.
Performance benchmarks show that Llama 3.2’s smaller models outperform peers in their class, and its larger models compete with closed systems like GPT-4. Overall, Llama 3.2 is a milestone in the evolution of edge AI, offering developers customizable, high-performance solutions for real-world applications.
Unleash the Superhero in You with Meta’s Orion Glasses: A Glimpse into The Future of Augmented Reality
Another key announcement by Zuckerberg at Meta Connect is Orion, a groundbreaking pair of augmented reality (AR) glasses that he touted as “the most advanced glasses the world has ever seen.”
Orion, which has been a decade in the making, features compact design, tiny projectors for a heads-up display, and neural interface technology that allows users to control AR experiences through simple gestures and thoughts, thanks to Meta’s acquisition of CTRL-labs. Positioned as a successor to Meta’s Ray-Ban Meta smart glasses, Orion offers a wider field of view, immersive AR experiences, and personalized AI assistance, all within an everyday wearable form.
Initially available to developers, Orion promises to push the boundaries of AR by merging digital and physical realities, offering use cases from real-time navigation to life-sized holograms. Meta also aims to build a new ecosystem for AR technologies.
Beyond Transformation: How dbt Labs is Shaping the Future of Data Democratization and Collaboration
At Big Data London, I spoke with Jeremy Cohen, Principal Product Manager at dbt Labs, about their recent innovations aimed at expanding beyond their core SQL-based data transformations.
While dbt has long been a leader in data transformations through its open-source dbt Core framework, the company is now focused on democratizing data access and fostering collaboration between technical teams and business users. One key addition is dbt Explorer, a tool in dbt Cloud that allows non-technical users to visually explore data, enhancing collaboration across teams. By addressing the persistent gap between data experts and business stakeholders, dbt Labs ensures business users are active participants in shaping how data is structured and analyzed.
Their platform now includes features like data quality checks, collaborative workflows, and visual exploration. In short, dbt Labs has evolved into a comprehensive solution for modern data teams. Looking forward, dbt Labs aims to remain the standard for data transformations while inviting broader participation from business domain experts, ultimately driving better decision-making and innovation.
GenAI (LLMs vs. Foundation Models): Difference Explained in Simple English
We explored the distinction between Large Language Models (LLMs) and Foundation Models, both commonly used in AI and natural language processing.
LLMs, such as GPT and BERT, are a subset of Foundation Models specifically designed for language-based tasks like text generation and translation. They excel at understanding language structure, adapting to new tasks with minimal data, and fine-tuning for specific NLP applications.
On the other hand, Foundation Models are broader AI models pre-trained on diverse data, enabling them to perform a variety of tasks beyond language, including vision and robotics. The key difference lies in their scope: while all LLMs are Foundation Models, not all Foundation Models are limited to language — they are general-purpose models adaptable to various domains.